Part 4: Emerging AI Technologies and Their Business Impact
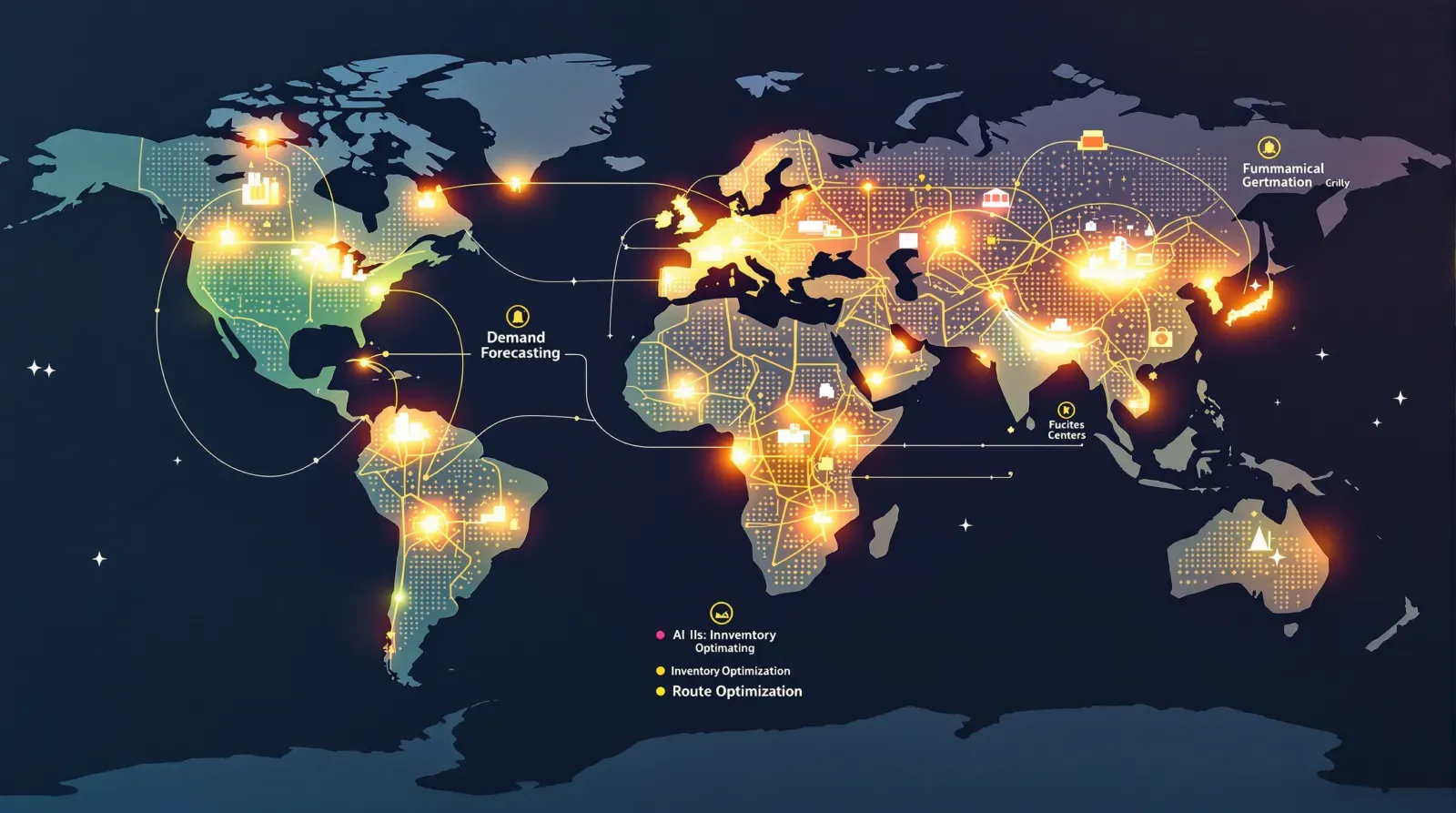
Introduction
Artificial intelligence (AI) is a rapidly evolving field, and 2025 is seeing the emergence of powerful new technologies that are poised to transform businesses. This post explores some of the most impactful emerging AI trends and their potential to revolutionize operations, decision-making, and innovation. We'll move beyond the theoretical and focus on practical applications and strategic considerations. For businesses ready to embrace these cutting-edge technologies, AI Startup LaunchPad provides the resources and guidance you need to succee
1. Multimodal AI: Beyond Single Data Streams
Multimodal AI represents a significant advance, integrating multiple data types (text, images, audio, video, sensor data) to create a more comprehensive and human-like understanding.
- What it is: Instead of processing data in isolation, multimodal AI systems can analyze and correlate information from different sources.
- Why it matters: This leads to richer insights, more accurate predictions, and more sophisticated AI applications.
- Business Applications:
- Enhanced Customer Service: Analyzing both the text of a customer's message and their tone of voice (through audio analysis) or facial expressions (through video analysis) to provide more empathetic and effective support. This could involve integrating tools like Beyond Verbal (for voice analysis) with existing customer service platforms.
- Improved Product Development: Combining customer reviews (text), product usage data (numerical), and user-submitted images/videos to gain a holistic understanding of product performance and areas for improvement.
- Advanced Security Systems: Combining video surveillance with audio analysis and sensor data to detect and respond to security threats more effectively.
- Personalized Marketing: Creating highly targeted marketing campaigns by analyzing customer interactions across multiple channels (website, social media, email, etc.).
- Key Players: While large companies like Google (with Gemini) and Meta are investing heavily in multimodal AI, the open-source community is also making significant contributions. The availability of pre-trained models and frameworks on platforms like Hugging Face makes it increasingly accessible to startups.
2. Small Language Models (SLMs): Efficiency and Specialization
While large language models (LLMs) like GPT-4 have captured much attention, small language models (SLMs) are gaining traction for their efficiency and ability to be customized.
- Advantages of SLMs:
- Lower Computational Costs: SLMs require less processing power, making them more affordable to run and deploy, especially for startups with limited budgets.
- Faster Inference: Their smaller size often leads to faster response times, crucial for real-time applications.
- Edge Deployment: SLMs can often run on edge devices (smartphones, IoT devices) rather than relying solely on cloud servers, enabling offline functionality and reducing latency.
- Specialization: SLMs can be fine-tuned for specific industries or tasks, often outperforming larger, general-purpose models in niche applications.
- Data Privacy: Running SLMs locally can address data privacy concerns.
- Business Applications:
- Industry-Specific Chatbots: SLMs trained on specific industry terminology and data can provide more accurate and relevant customer support.
- Content Moderation: Tailored SLMs can more effectively identify and filter inappropriate content for specific platforms.
- Medical Diagnosis Assistance: SLMs trained on medical literature can assist healthcare professionals.
- Financial Analysis: Specialized SLMs can process financial documents and provide tailored insights.
- Examples: Mistral AI's models, Meta's Llama 2, and models available through platforms like Hugging Face offer a range of options for businesses looking to leverage SLMs.
3. Retrieval-Augmented Generation (RAG): Bridging the Knowledge Gap
Retrieval-Augmented Generation (RAG) combines the creativity of generative AI with the factual grounding of information retrieval, resulting in more accurate and reliable AI outputs.
- How RAG Works:
- Retrieval: When a RAG system receives a query, it first searches a knowledge base (e.g., a database of documents, a company's internal knowledge repository) for relevant information.
- Generation: The retrieved information is then used along with the original query as input to a generative AI model (like an LLM), which generates the final response.
- Benefits for Businesses:
- Improved Accuracy: By grounding the generation process in factual information, RAG reduces the risk of AI "hallucinations" (generating false or misleading content).
- Enhanced Contextual Understanding: RAG allows AI systems to access and leverage up-to-date information, leading to more relevant and contextually appropriate responses.
- Customizability: Businesses can use their own proprietary knowledge bases to tailor the AI's responses to their specific domain and expertise.
- Reduced Training Costs: RAG can sometimes achieve good performance with less training data than purely generative models.
- Business Applications:
- Customer Service: RAG-powered chatbots can provide more accurate and informative answers to customer questions by drawing on a company's knowledge base.
- Content Creation: RAG can help generate more factually accurate and well-researched articles, reports, and marketing materials.
- Research and Development: RAG can assist researchers by quickly finding and summarizing relevant information from vast scientific databases.
- Legal and Compliance: RAG systems can help legal professionals quickly retrieve and apply relevant case law and regulations.
Ready to implement RAG and other advanced AI techniques? AI Startup LaunchPad provides the guidance and resources you need to get started.
4. Digital Twins: Connecting the Physical and Digital Worlds
Digital twins are virtual representations of physical objects, processes, or systems, updated in real-time with data from sensors and other sources. They offer powerful capabilities for monitoring, analysis, simulation, and optimization.
- Key Features:
- Real-Time Data Integration: Digital twins are constantly updated with data from their physical counterparts.
- Predictive Capabilities: By analyzing data, digital twins can predict future performance and potential issues.
- Simulation: Businesses can test scenarios and changes in a virtual environment before implementing them in the real world.
- Optimization: Digital twins can suggest improvements to their physical counterparts.
- Business Applications:
- Manufacturing: Digital twins of production lines can optimize processes, predict maintenance needs, and improve quality. Siemens is a major player in this space.
- Healthcare: Patient digital twins can aid in personalized treatment planning.
- Urban Planning: Digital twins of cities can assist in traffic management and energy optimization.
- Aerospace: Digital twins of aircraft engines can predict maintenance needs.
- Retail: Digital twins of supply chains can optimize inventory management.
- Construction: Bentley Systems
5. AI-Driven Decision Making: From Insights to Action
AI is moving beyond providing insights to actively participating in decision-making processes.
- Evolution of AI in Decision Making:
- Increased Data Processing: AI can analyze vast datasets, including unstructured data.
- Advanced Predictive Analytics: AI can make accurate predictions about future trends.
- Real-Time Analysis: AI can process data in real-time, enabling immediate decisions.
- Integration of Multiple Data Sources: AI can combine data from various sources.
- Key Benefits:
- Enhanced Accuracy: AI can process data at a scale and speed impossible for humans.
- Reduced Bias: Well-designed AI systems can help mitigate human biases.
- Faster Decision Making: AI provides rapid analysis and recommendations.
- Improved Risk Management: AI can identify potential risks and simulate scenarios.
- Business Applications:
- Strategic Planning: AI can analyze market trends and competitor actions.
- Financial Management: AI can optimize investment portfolios and predict cash flow.
- Supply Chain Optimization: AI can analyze data to optimize inventory and predict disruptions.
- Human Resources: AI can assist in hiring decisions.
- Product Development: AI can analyze feedback and market trends to guide development.
- Challenges: Data quality, ethical considerations, human oversight, and integration with existing processes are crucial.
Conclusion: Embracing the AI-Powered Future
The emerging AI technologies discussed in this post – multimodal AI, small language models, retrieval-augmented generation, digital twins, and AI-driven decision making – represent the cutting edge of innovation. These technologies are not just theoretical concepts; they are being implemented now by forward-thinking businesses.
The key to success is a balanced approach that combines the power of AI with human expertise. Businesses that can effectively leverage these technologies while addressing the challenges will be well-positioned to thrive.
Looking to build a future-proof business powered by the latest AI innovations? AI Startup LaunchPad provides the roadmap and resources to help you succeed.
Call to Action:
- Identify opportunities: Determine which emerging AI technologies are most relevant to your business.
- Research and experiment: Explore available tools and platforms, and start with pilot projects.
- Develop a long-term AI strategy: Plan for how you will integrate these technologies into your operations and decision-making processes.
- Invest in training: Ensure your team has the skills and knowledge to work effectively with these new AI tools.
- Prioritize ethical considerations: Implement responsible AI practices from the start.
Ready to take the next step? AI Startup Launchpad can be your guide.